AI Spotlight
PREDICTING LOADING, TRAVEL, AND UNLOADING TIMES IN READY-MIX CONCRETE DELIVERIES WITH AI
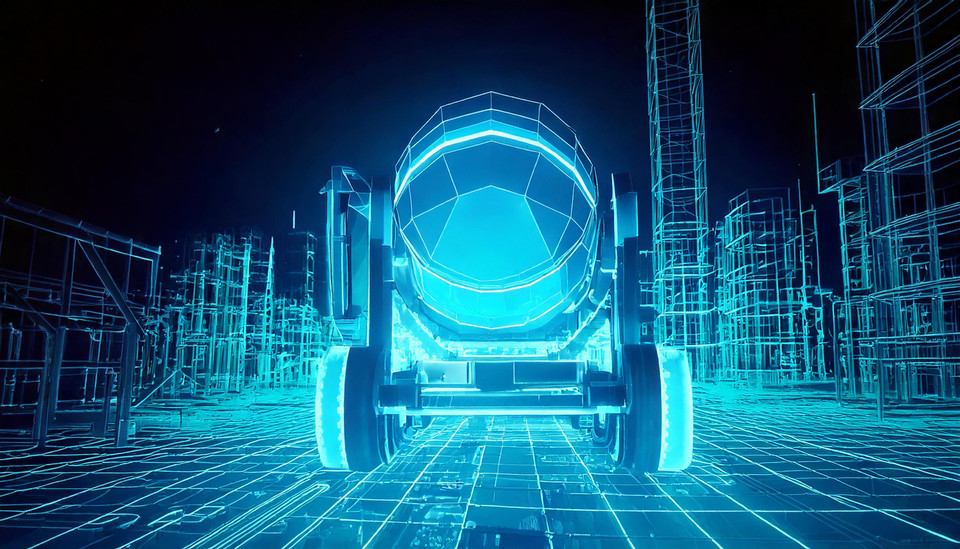
Ready-mix concrete is a perishable product. It needs to be delivered to the construction within 90 minutes. After this time, the mixture starts to harden, making it difficult to pour. Explore how Machine Learning (ML) can help make better predictions for loading, travel, and unloading times of ready-mix trucks.
Key Benefits
- Increased Accuracy: Significantly improves the prediction accuracy of unloading times at construction sites.
- Cost Savings: Reduces operational costs through better truck fleet utilization.
- Carbon Footprint: Reduces concrete waste and CO2 emissions through less truck idling.
Use Case
Summary
- Any planning tool based on Operations Research (OR) is only as good as the quality of its input data. Bad inputs will result in bad outputs. As such, we undertook an assessment project looking at how Machine Learning (ML) could be used to improve the quality of input parameters that influence the optimization calculations of our transport planning software.
How It's Done
- As a first step, we took a closer look at loading, travel, and unloading times.
- We analyzed the data of 150,000 ready-mix deliveries and compared our expected loading, travel, and unloading times against the actual values.
- The next step was to look for the most relevant variables that will help to achieve more accurate predictions, using the so-called ‘random forest approach,’ a widely applied and proven ML approach to rank the importance of variables in a regression problem.
- Having done all analyzing and modelling, we put the new model to a real-life test. This was our test ground: 40 ready-mix plants, five consecutive planning days, on average 275 trucks making 1,060 deliveries a day to an average of 350 customer locations, supplying almost 10,000cbm of concrete.
Did You Know?
- We managed to estimate the average loading time by 1:23 minutes more accurately, while the average travel time results were six seconds more accurate. The biggest gains in accuracy were, as expected, in unloading times: 8:45 minutes.
- Or in other words, for a delivery that takes 2 hours and 10 minutes in total, we were able to get our planning calculations almost 10 minutes closer to reality.
Interested in exploring more AI Spotlights?