Feb 25, 2020 Roy Prayikulam
ShareHeader Photo: GettyImages/Own Creation
Machine learning is nothing new. In fact, many of the algorithms used to make predictions have been around for decades. Nevertheless, there is a current hype around the topic of Artificial Intelligence and Machine Learning that spans across nearly every industry, and banking is no exception.
One reason for the increased interest in machine learning is the expanded availability of data. Every transaction can now be supplemented with card information, IP and device data, merchant information, user behavior, delivery addresses, known fraud patterns and much more. However, manually analyzing all this data in a split-second to make a decision about whether it is fraudulent, is not humanly possible. A digital decision-making engine is therefore a must, and by integrating both best practice, knowledge-based rules with data-driven methods, this engine can become a powerful fraud and financial crime-fighting solution.
To clarify, knowledge-driven methods are based on human intelligence and expertise, for example fuzzy logic, statistical profiling, scorecards and other mathematical algorithms. Data-driven methods include data mining and machine learning. Machine learning models require ample data to be able to learn accurately and the necessary volume of data may not always be available, especially with new platforms. This is one of the reasons why the knowledge-based approach simply cannot be disregarded and replaced by machine learning. Combining the best human intelligence-based AI and machine learning technology into a single solution sums up the Hybrid AI financial crime fighting strategy.
Before jumping into a machine learning project, it is of utmost importance to define your goals and determine what questions are being addressed. What are the prediction goals and is there enough data to feed to the machine? The next step is to start preparing the data. In a typical machine learning project, approximately 80% of resources will be invested into data preparation, which encompasses data transformations and feature engineering. Machine learning-ready data can then be split into training and testing sets and a suitable model chosen, commonly either a supervised or unsupervised model.
With supervised learning, the data provided to the machine for learning purposes has clear labels. For example, transaction data can be labeled either as fraudulent or legitimate based on feedback from customers and investigation results. This information is passed on to the machine which seeks commonalities across these labels so fraud predictions can be made and applied to future transactions. In unsupervised learning, the input data does not have labeled responses - instead clustering and anomaly detection algorithms are used to identify suspicious activity. For instance, imagine that a merchant applies for an account at a bank as a bakery. When assessing the application, the bank can take data from other bakeries and feed it into the machine to search for any unusual activities or behavioral patterns for the new merchant, compared to other bakeries. This approach can highlight application fraud, and can also prove useful for AML compliance topics such as ongoing Customer Due Diligence.
Evaluating a model using a test data set is an important next step. This helps assess performance before exporting the model to real-time production. The move to a real-time engine is often a stumbling block for financial institutions. It is one of the key steps we simplify for our clients with our RiskShield Machine Learning (ML) solution.
When it comes to integrating machine learning into fraud and financial crime fighting efforts, it is important not to try and tackle everything at once. Start with a specific area of business, such as fraud in cards payments or internet banking, and slowly expand after experiencing success. It is an iterative process that should not be underestimated.
RiskShield ML provides everything needed for a smoothly functioning Hybrid AI approach, encompassing both knowledge-based and machine learning methods. The RiskShield Machine Learning environment is used to create and test models that can be implemented in real-time within the RiskShield decision engine. These models can be used to supplement the scorecards, statistical profiling, fuzzy logic and other knowledge-based methods. This results in fewer false positive alerts and enhanced detection of new modus operandi, which increases accuracy. The predictive models used within RiskShield Machine Learning also come with interactive visualization features that make them easy to interpret.
This article was originally published in Payments Cards & Mobile: "2020 Fraud and Financial Crime Report":
Click here download the full report
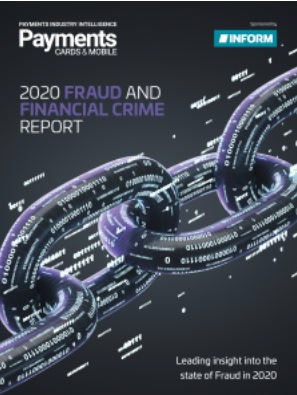
About our Expert
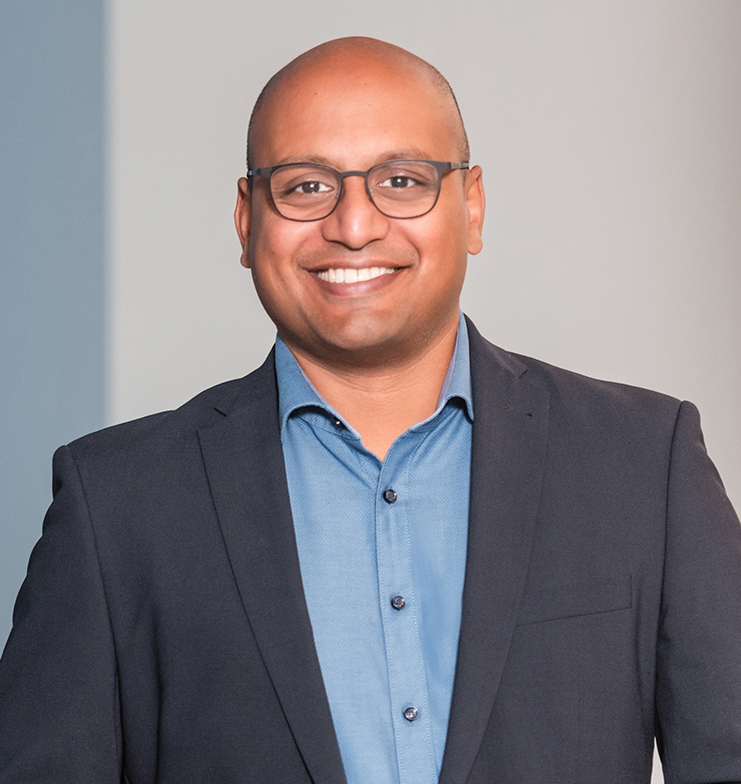
Roy Prayikulam
Senior Vice President Risk & Fraud
Roy Prayikulam is Senior Vice President Risk & Fraud at INFORM. He has significant experience working in complex IT integration projects for the financial sector, such as acquiring, card issuing, Internet banking, and compliance. He graduated from the RWTH Aachen University in Business Administration, Computer Science, and e-Business.